Naming in an A.I. Age- Exploring the Intersection of AI and Market Research
Introduction
The integration of artificial intelligence (AI) into various aspects of our lives has been a topic of discussion for many years. Since the public release of ChatGPT in November, AI has garnered even more attention. In this podcast transcript, Mike Carr and Jian Huang engage in a compelling conversation about the role of AI in market research, its limitations, and the future of data-driven decision-making.
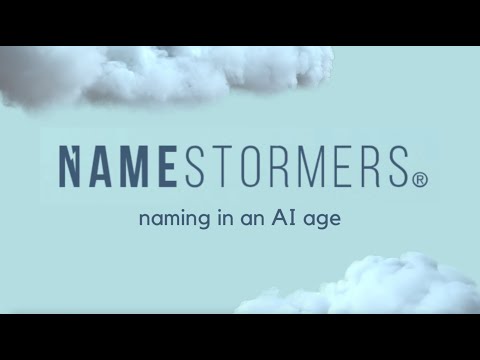
Exploring the Intersection of AI and Market Research
AI in Market Research: A Blessing or a Threat?
Mike Carr opens the discussion with a pertinent question: will AI replace traditional market research and name testing? Jian Huang, a computer science researcher, provides insightful perspectives:
- Data-Intensive AI: AI’s recent breakthroughs primarily revolve around data-intensive AI. While earlier AI approaches focused on reasoning and logic, data-intensive AI, like ChatGPT, relies heavily on extensive data.
- ChatGPT’s Strength: ChatGPT excels in reading and recalling information from a vast database, making it an impressive data processor. It can appear to perform calculations because it has seen similar data in documents.
- The Role of Human Creativity: Despite AI’s data processing prowess, the thinking and creativity remain in the hands of human researchers. Humans decide what perspectives matter the most and how to interpret data. AI serves as a tool to enhance human capabilities.
- Name Testing and Originality: AI can be valuable for tasks that involve processing large volumes of data. However, it struggles when dealing with entirely new concepts or original ideas. In name testing, if a name is entirely unique and unseen before, AI may not provide valuable insights.
The Challenge of Synthetic Respondents
The conversation shifts to the idea of using synthetic respondents in research. The concept of creating artificial respondents that mimic human behavior and reactions to certain stimuli is discussed. Huang and Carr express skepticism about the feasibility of using synthetic respondents in market research, especially in domains where the nuances of human social behavior play a significant role.
The Vision of Mapprio and the Future of Market Research
Jian Huang, the founder of Mapprio, shares insights into the future of market research:
- Unlocking Data’s Value: Huang believes that there is untapped potential in data. Many valuable insights remain hidden due to a lack of time and expertise. He aims to develop tools and platforms that empower users to extract the full value of data, particularly for tasks like micro-segmentation.
- Human Collaboration: Huang recognizes that data is more than numbers; it’s intertwined with the context in which it’s collected and used. He values the collaboration with industry experts who provide context to data.
Conclusion
The conversation between Mike Carr and Jian Huang highlights the dynamic landscape of AI in market research. While AI offers powerful data processing capabilities, its limitations become apparent when faced with originality and the subtleties of human behavior. The future of market research is likely to involve a harmonious blend of AI tools and human expertise, with a continued focus on unlocking the untapped potential of data.
Transcription:
Mike Carr (00:04):
AI has been talked about since November when they released ChatGPT in the general public. It’s been talked about for decades before that we’ve gone to some AI conferences. I’m sure you have too. The big concern, and this is probably overstating it, but is AI going to replace how we do market research and name testing and if so, how? Or if part of it’s going to be replaced, how and if not, where do you not think AI, LLMs, all this other stuff is really going to make an impact? What are your thoughts on that?
Jian Huang (00:40):
Oh wow. How long is your show? Three hours?
Mike Carr (00:44):
Yeah. Yeah.
Jian Huang (00:46):
This is a long topic. So first off, as someone who teaches and does researching computer science and of course funded by federal agency to do computer science, so my view is probably a little different from the general public. So first off, I don’t see AI as a threat at all and here are my reasons. So the AI that has gotten everybody’s attention, those are data intensive AI, I really want to emphasize this. It is data intensive AI. So there are other kinds of AI that was done years before that really wanted to do reasoning, logic and all of that without a whole lot of data. Those didn’t go anywhere and that’s why you didn’t hear about them. Now suddenly we’re now hearing about this data intensive AI. Then what does it depend on? What data? Lots and lots of data. So what ChatGPT is good at is it can read faster than anyone can and it actually remembers everything better than everybody can.
(01:55):
So in that regard, it is better than any human and to a degree where it can even appear to do math. But here’s how it works. Chat GPT is good at inferring what the next word should be. It can consider a very, very big context and how it first is simply this. I’ve seen that before somewhere in certain document that I’ve seen and I’ve seen that more than once. So I believe it pretty deeply and put it out there. That’s how that works. And so if you are reading press, you should be impressed by a eight year old kid who can read everything, remember everything and regurgitate everything. And you shouldn’t be threatened by the eight year old how it appears to do math such as say five plus three equals eight. So it’s because it has seen it in some documents before.
(02:51):
Now go to ChatGPT and try the following. Try 34511.375 plus two. It cannot do it because in that case I don’t think it has seen that in any document in the past. So due to that reason then if we look at the market research, so if you are needing to read a lot of documents in that case ChatGPT is probably pretty good. But I really want to say for a researcher, this is almost like you’ve got an intern who has this eight year old ability to memorize everything. The thinking part is still up to you and choosing what perspective matters the most is up to you. So I don’t see a threat there. I see a productivity boost in name testing. I really want to say I don’t see AI replacing name testing at all because if it’s a new name, the word has not seen it before or not in the context in the business context they’re talking about.
(03:58):
So there is nothing to read, nothing to memorize ChatGPT can’t tell you that, hey, I’ve seen this before. Now in that case ChatGPTcan do one more thing that the general public would call hallucination because I think I’ve seen something else that’s close enough and I can piece it together. Looks good enough to me. So in that case, that’s where the hallucination comes from. But it’s not like there is a doctor evil behind trying to piece things together. It’s all because I’ve not seen it before. So then testing, if it’s all new and it’s on the leading edge, there’s no way data intensive AI can help. There’s no data data.
Mike Carr (04:42):
We have seen that in a lot of ways. So if it’s a traditional industry where there, let’s say banking and there are a ton of banks out there and there has not necessarily been a lot of innovation, at least up until fairly recently, and we use ChatGPT with the right engineer, prompt engineering, it’ll come back with names that sound good for what’s been out there, right? They’re familiar, they’re sort of, yeah, that sounds like a bank name. But as soon as you start introducing because there’s a lot of data, there’s a lot of banks and a lot of names out there, and so it’s easy to infer that. But as soon as you say, well, this is a different kind of bank, this is a bank that has an ITM, not an ATM, well there’s some question, does a ChatGPT have enough data even about what an ITM is versus an ATM to even know what you’re talking about?
(05:33):
And then it also has, it’s a hybrid model, so it’s not just a standalone ITM. We’re going to have a bunch of these ITMs, these interactive technology terminals where you can do a lot of the banking with the help of tech. We’re also going to have somebody that’s live that’s on site that’s able to help you use that tool. So it’s this hybrid of, well, we’re going to have this new kind of branch that’s mostly tech driven, but there’s also real life people there to help you. There’s no way in the world we’ve seen and we’ve tried, they can’t come up with anything that’s exciting and new. It just comes back with a lot of the same stuff. And of course a lot of the same stuff is not only boring and is not only on brand or on message, but it has all kinds of trademark problems. It sounds like everything else has already been tried over and over
Jian Huang (06:22):
And So every university has a AI task force right now because everyone is scared that by essays now being produced in seconds. So I’ve been part of that conversation too, but one thing that’s really interesting is sometimes when faculty get frustrated, we joke with each other. For example, to say you see chat GPT can replace one thing really, really well. That is mediocre writing.
Mike Carr (06:57):
Yes.
Jian Huang (06:58):
So if you want something average, something normal looking, something that fill the space ChatGPT is really, really good. But if you want anything original, I just don’t see it. Don’t see it.
Mike Carr (07:09):
That’s very common.
Jian Huang (07:10):
So if you have a assignment for students where you demand some originality where you think you’ve taught things something original and they can do it, if the results come back is so mediocre, you can actually tell you don’t even need to send it into a cheat checker in names, especially with the kind of work that you guys do. I really think those are original compelling, sometimes two thought provoking where I want to react to saying, Hey Mike, what is that? But nothing that’s like the normal thing out there. So I guess just I have to concede a little bit mediocre name and all of the business operation around that may be doable by ChatGPT maybe. But if you’re on good original compelling things, maybe whatever ChatGPT says don’t do.
Mike Carr (08:06):
Okay, so I have a question that I think I know you’re going to answer to, but it was the subject to much conversation in one of the last market research conferences I attended, and that is the synthetic respondent that there is a lot of data about individuals and how they react in certain situations. And so the presentation was around you can do a study, a name testing study or any other kind of name testing study, but instead of using real life people, you sort of specify the demographics or the psychographics like, hey, I need 500 gals between 18 and 35 making
Mike Carr (08:48):
This much money with three kids and they live at home. They don’t have, I mean whatever those parameters are, and it supposedly is going to be able to evaluate as if they were real people and come back not just with the quant data but with the open end verbatims, right? The actual reasoning, the rationale. Now I don’t see how that really is going to work, but I didn’t know if you had a point of view on that. Once you’re thinking about in the future, do we really think you’re going to be able to conduct a study with a robot or a synthetic respondent as opposed to a real human being?
Jian Huang (09:27):
Well, so not speaking as a computer science person, just speaking as a person, I’ll say over so many years, centuries humanizing has been a good thing on that notion, the idea of creating synthetic respondents so that we don’t have to have humans anymore. I’m just philosophically against it. And then as a computer science person, let me say this, if it is a use case that is so well understood, there’s so much data behind it, yes, we can build a software agent that can learn from all of that and be really, really good. And certain situation actually needs this like self-driving car. And that’s exactly that. And there are other situation where for example, right now there are AI agents that can diagnose e e G very well. There are AI agents that can diagnose a tumor really, really well. And those are all great things because in that case it’s a specific nut task and the machine is indeed better than a human. But to build a bunch of robots that pretend to pretend that the use case that you have is so well understood to every bit of nuance. I think that’s scientifically too far a stretch. And I want to stay on the academic language, academic language side, but I think it’s stretching the fact and stretching the capability.
Mike Carr (11:17):
I think that’s a great comment. I mean we’ve done some testing where we actually will try chat GPT or a bard or whatever, and then we’ll test it the real way, right? With real people or with hard data. And
Jian Huang (11:31):
What
Mike Carr (11:31):
Scares us to death still is that hallucination factor that you mentioned, that you have to know enough about your domain, your subject matter, and be expert enough in it to ascertain when what you’re getting from AI is helpful and is going to compliment and is probably pretty close to the truth. Like a tumor that’s a certain size for a certain age segment or whatever
(11:57):
Versus something that’s just fabricated. And we’ve seen some fabrication, it sounds great. It sounds like, oh yeah, this root means this awful thing in this culture, in this language. And you go out and you check it and it doesn’t mean that at all. People that have lived in that culture, that speak that language have no clue where. And so I think it’s going to be helpful in those areas where you have the expertise and knowledge to really vet the answer and use it the right way as a compliment and a supplement. But I do think that in those domains where you can’t check it, it’s like move forward at your own risk, right? There’s a big danger sign with the skull across bone. Don’t go into that swamp because you’ll never get out.
Jian Huang (12:42):
So right now, the research that we are doing are aiming to build AI agents that are assisting human on particular tasks. Those methods are really task oriented. Now let me tell you why that approach will never translate to human side. It’s because humans are social beings. The motives within humans are social related. You can say it’s your private thought, but it is for social reason. And if we really think about it and self-check that is the case and to such a point where if we say our social identity is how we define ourselves, then it’s not just a relationship a motive. It is how we define ourselves. Then in that case, unless you truly understand the social surroundings of the testing subject, I really want to say you should not assume there is any data to help you understand how they actually think knowing their age and gender means nothing. They’re social beings to begin with. So I really believe the name testing stuff you guys are doing are the ones that are, I’m going to say the gold standard. I don’t want to say there will be never anything better, but I see you guys as the gold center right now.
Mike Carr (14:08):
Well, and it is tough to mimic that real world, right? I mean, we try to show a stimulus, like let’s say it’s a physical product. Well, we feel pretty strongly you want to try to present that stimulus in as real world a setting as possible. So you drop the name on the package with minimum text or words that you require the respondent to read because they’re not going to read it in a real world situation anyway, or a video or whatever that stimulus is to try to say, look, no name is ever out of context. Even in conversation, there’s
Mike Carr (14:42):
Some context that wraps around that name and provides some ideas to what you’re talking about, what the social context is, what the product or service offering is, and you cannot ignore that. And I think that’s going forward, going to become even more important. And so talk about going forward, map Rio and you as the founder of Map Rio, you’ve probably got some plans for how that platform’s going to evolve. I would just be interested in anything else you want to talk to us about why you built Map Rio and you’ve shared some of that already, but perhaps more importantly for our listeners where you see the future and where you want to take market research and the kinds of things that you’re doing to that next level or that next couple levels. Any thoughts or any vision you can share with us without revealing anything that’s proprietary?
Jian Huang (15:34):
Oh, well actually, Mike, in this case I want to say working with industry leaders like you actually taught me how a lot of things work. So I want to thank you for that overall vision. Here’s one thing I can say, and I think many people will agree with me. Data itself is relatively new in human civilization for many, many years without knowledge are embodied in books. Data itself is an embodiment of fill in the blank. You can say insights, you can say knowledge, you can say power, or you can even put a dollar sign there. However, the ability to work with data is not widespread. And so in this case, a question that we often see people ask is, do you think I’ve gotten everything out of the data already? And our answer usually is no, you’re just question surfaces. So this ink interesting dynamics leads us to see the following as a huge opportunity that is, there are plenty of things that you can do with data you’re not doing because you don’t have time and not just you don’t have that expertise because there’s so many self-learning things out there.
(17:01):
Now, if you want to pick up the skill you can, right? So I want to say lack of time is the key due to lack of time. You actually don’t do those important things with the data, hence you don’t get to see all everything the data can offer doing micro segmenting happens to be one great example of that. So one vision that we have is to create as much tools as we can to put all of these values that can be extracted from data into our user’s hands. Things that they couldn’t do just because they didn’t have time, not because they didn’t know they’re valuable. So due to that, we have created a suite of data products and you guys have been using a lot of those. I’ve heard good things from you guys. Thank you. And then so build action priorities grid, and we build complete profile and we want to build more. So that’s the overall vision and that’s what the mission that Map Rio is on.
Mike Carr (18:01):
Awesome. So if one of our listeners wanted to find out more about you and Map Rio and where you’re going and how they might be able to take advantage of your platform, where would you like for them to go? How do they get ahold of you?
Jian Huang (18:16):
The easiest is LinkedIn. I have, due to many reasons, spent a lot of time on LinkedIn and now I’m a little addicted. I’m an addict. So the easiest is on LinkedIn. And also full disclosure, my name is like a John Smith in English. So there are many, many Gen Huangs out there in this world. So I’m the one that’s at University of Tennessee, so that’s pretty easy to find. And also go to map real website, that’s an easy thing. But I’d love to connect with people, learn from our users. And one thing I realized is I really understand data, but until you understand the context of how data is collected and how data are used, even, I don’t know how to extract the full value of the data. So meeting you, Mike, meeting Kay has been very valuable to me. So if I can connect with more people on LinkedIn, I’d love to.
Mike Carr (19:23):
And that’s great. And even though you’re a professor at the University of Tennessee and not University Texas,
Jian Huang (19:29):
Don’t hold that against me
Mike Carr (19:29):
Texas here in Austin, we’re glad that we have this relationship. So just for our listeners, the website is map MAPPRIO.com. And Jian, could you just spell your name for anyone that wants to try to find you? So they’ve got the spelling correctly.
Jian Huang (19:51):
Okay, so it’s just about map. Map. The name comes from mapping priorities. That’s how the name came about. So map priorities Mapprio. My name is Jian Huang, JIAN– on certain days, it could sound like a certain alcohol drink. Last name is Huang, HUANG. And I’m not related to the CEO of Nvidia.
Mike Carr (20:22):
Oh, too bad.
Jian Huang (20:23):
Not at all.
Mike Carr (20:24):
Not all. No stock options there
Jian Huang (20:27):
Very, very far. 500 years ago the family may cross the branch a little bit. Yeah, but not related.
Mike Carr (20:36):
Okay. Well Jan, thank you so much for your time today. Great insights, great discussion. Really appreciate it. Wish you the best of success and have a great weekend.
Jian Huang (20:46):
Yeah, wish you guys the best too. Thank you for having me.